Proposal Defense: Ajimon Thomas
Presentation: HPC User Research Symposium
Wind and Plume Driven Circulation in Estuarine Systems
The study begins with an application of a state-of-the-art storm surge model to examine the effects of meteorological forecast errors on coastal flooding predictions along the North Carolina (NC) coastline. As Hurricane Arthur (2014) moved over Pamlico Sound, it increased the total water levels to 2.5 m above sea level; this water pushed first into the river estuaries and against the inner banks, and then moved eastward to threaten the sound-side of the barrier islands. It is hypothesized that a combination of storm track and intensity errors caused errors in the forecast winds and water levels along the NC coast during Arthur. Model results reveal that, as the forecast storm track and intensity errors increase, the errors in forecast wind speeds also increase, but the errors in forecast water levels remain relatively the same, signifying the non-linear response of the coastal ocean to wind effects. By separating the forecast errors in storm track and storm strength, this study quantifies their effects on the coastal ocean, which provides useful guidance for designing relevant forecast ensembles.
In addition to flooding impacts, storms can also cause dramatic changes in estuarine salinities, which can negatively impact estuarine ecosystems. Baroclinic models are useful tools for predicting estuarine salinity response under changing environmental conditions. In the present work, the features of wind- and plume-driven circulation in the vicinity of Choctawhatchee Bay (CB) and Destin Inlet, Florida, are analyzed with a recently-enhanced, three-dimensional, baroclinic model. Satellite imagery showed a visible brackish surface plume at Destin during low tide. The goal of the present study is to quantify variability in the plume signature due to changes in tidal and wind forcing. Modeled tides, salinities and plume signature are validated against in-situ observations and satellite imagery and then applied to analyze plume response in two scenarios. In the first case, model plume behavior is analyzed on successive days of near-constant tidal amplitudes and changing wind directions due to passing cold fronts. In the second case, plume response is investigated during consecutive days of neap-spring variability in the tides and near-constant wind speeds. Model results reveal a larger plume during spring tides and periods of weak wind forcing. Oshore winds enhance the north-south expansion of the plume, whereas onshore winds restrict the plume to the coastline.
Finally, the validated model is applied to identify salinity and transport characteristics within CB. Based on past studies, it is hypothesized that CB is a stratified system with limited flushing and zones of distinct salinity gradients. These hypotheses are tested by analyzing bay salinities from the validated model during a period of low river flows. Model surface salinities indicate brackish conditions (20 psu) throughout the bay except for near the river mouth. Stratification (10 to 15 psu) within the bay is unaffected by the passage of cold fronts and neap-spring tidal variability. The residence time within the Choctawhatchee Bay, an important indicator of estuarine health, is computed via particle tracking and is equal to roughly 40 days.
This work advances the scientific understanding of multiple aspects of estuarine circulation including wind-driven surge and flooding, brackish plume behavior through inlets and onto the shelf, and salinity transport and stratication properties within estuaries. Research ndings lead to a better understanding of estuarine response under a wide range of atmospheric conditions, and the resulting technologies will be useful for oil spill response operations, fisheries and pollution management.
Alireza wins Student Educational Award
Ph.D. student Alireza Gharagozlou won the Student Educational Award at the ASBPA National Coastal Conference 2018. This award is given annually to an undergraduate or graduate student who, through his or her research, is furthering the state of the science of coastal systems as it relates to the goals and mission of the ASBPA. Congrats to Alireza!
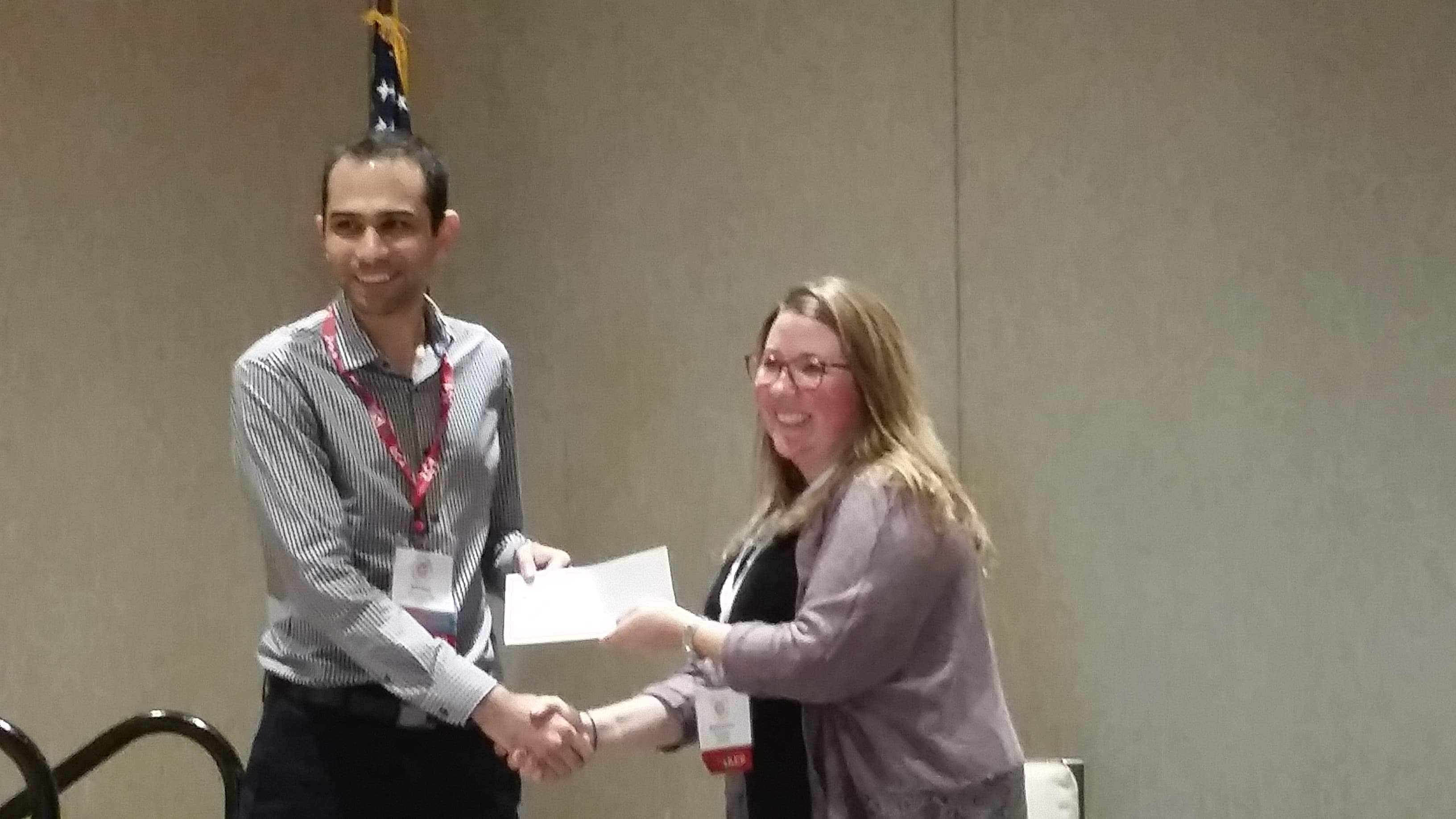
Alireza accepts the Student Educational Award during the awards luncheon at the ASBPA National Coastal Conference.
Conference: ASBPA Coastal Conference 2018
Celebration for Rosemary Cyriac
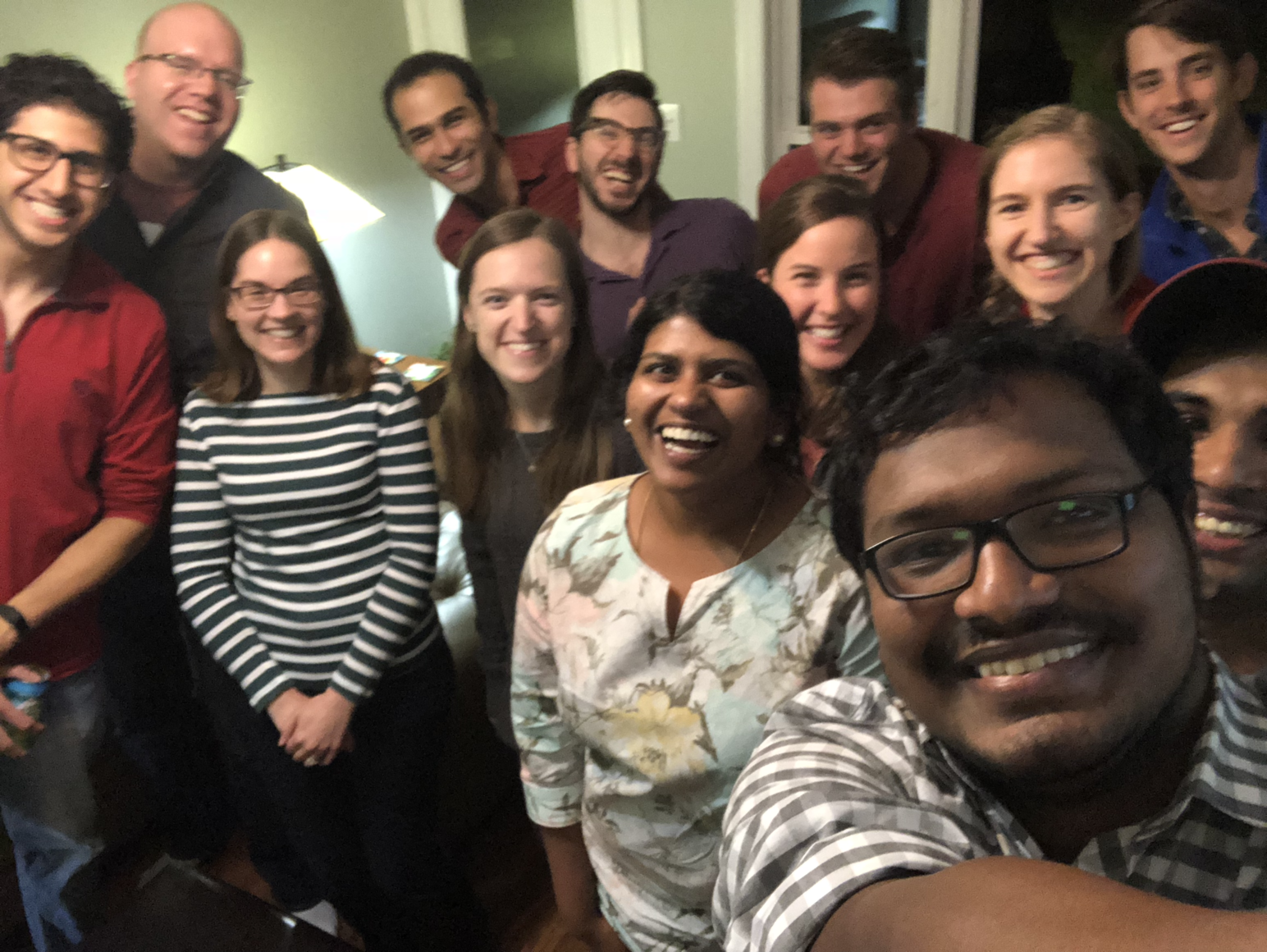
Members of the NCSU coastal engineering team celebrating the successful PhD defense by Rosemary Cyriac.
Seminar: Coffee & Viz
N Tull, JC Dietrich+, TE Langan, H Mitasova, CA Rucker, BO Blanton, JG Fleming, RA Luettich. “Enhancing Visualization of Storm Surge Guidance.” Coffee & Viz, NCSU Libraries, North Carolina State University, Raleigh, North Carolina, 19 October 2018.
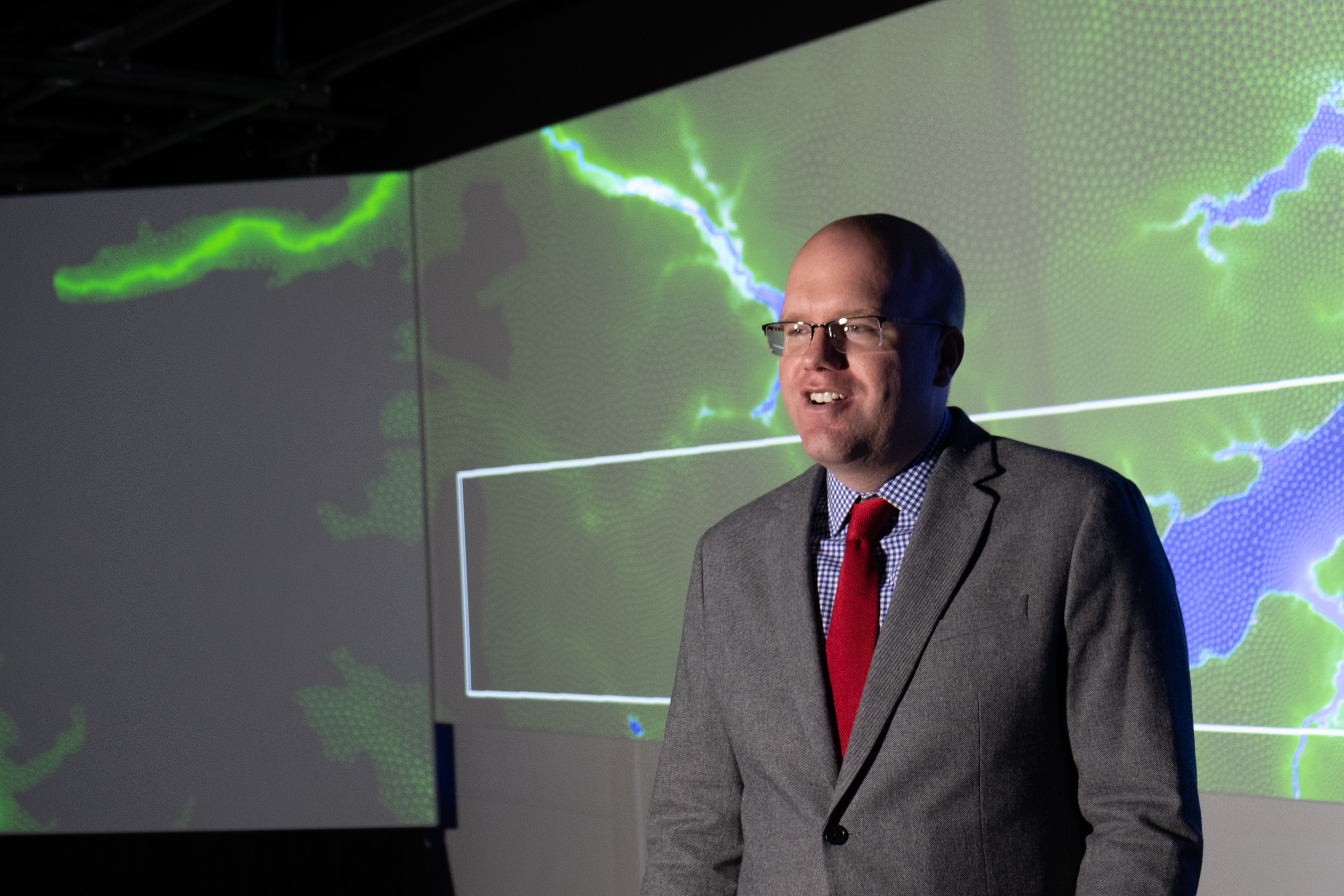
Casey Dietrich presenting at Coffee & Viz. Photo courtesy Julie Dixon.
This seminar was part of the NCSU Libraries’ Coffee & Viz event series, and it was held in the Teaching and Visualization Lab. This lab has 10 overhead projectors and can display graphics on three walls — the entire front and both full sides of the room. More photos are included below.