Category Archives: Models
Posters: Summer 2024 Conferences
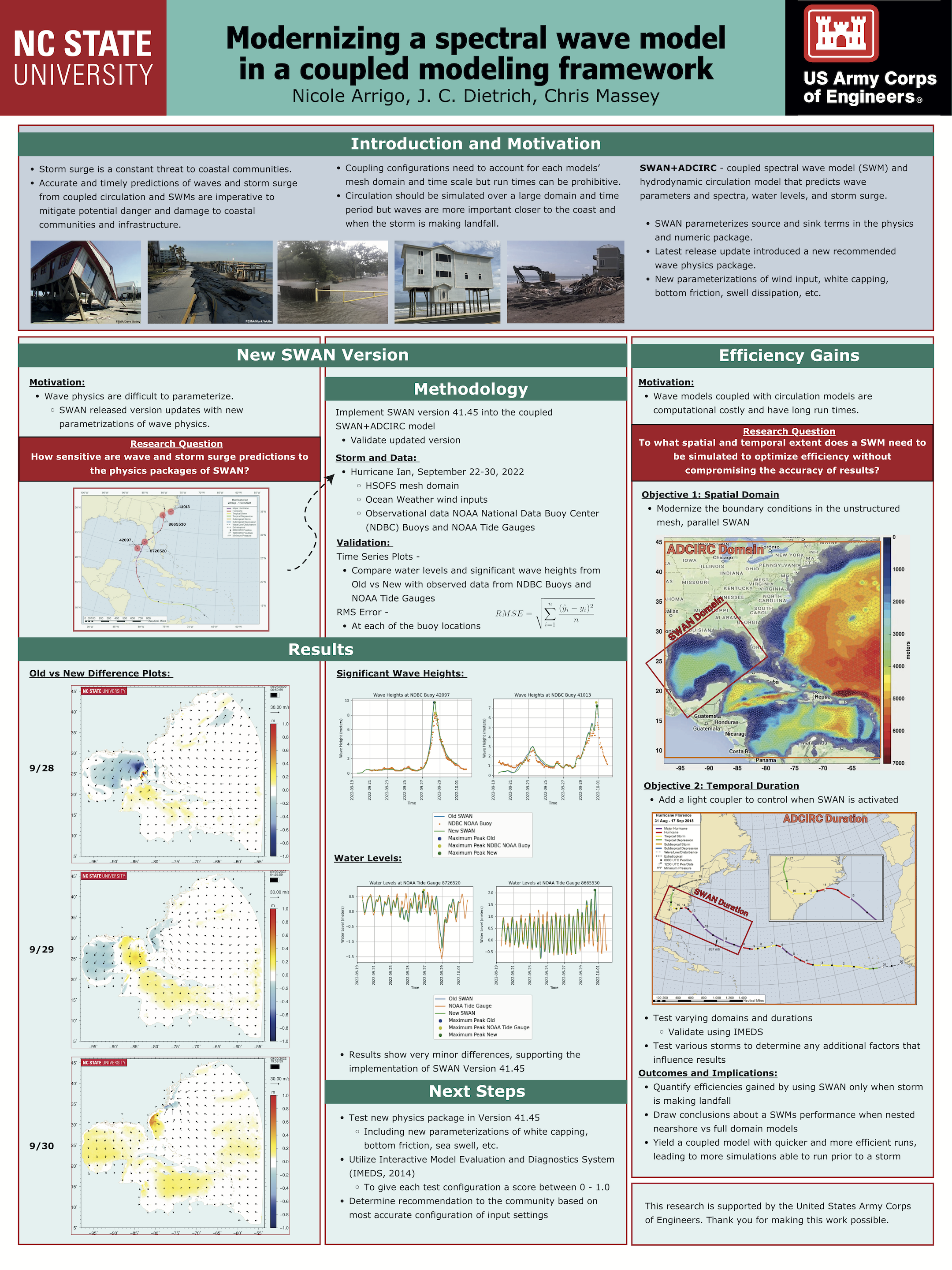
Modernizing a Spectral Wave Model in a Coupled Modeling Framework
Prediction of Peak Water Levels during Tropical Cyclones with Deep Learning
In this research, we implemented a neural network to predict peak values for total water level (tides and storm surge) at multiple stations, considering astronomical tides and storm tracks of any duration as inputs. To create the training library, we simulated 1,813 synthetic tropical cyclones based on historical data in the North Atlantic Ocean, with a specific focus on storms that affect North Carolina. These simulations used a full-physics hydrodynamic model with variable spatial resolution of about 50 m near the coast. The outputs were downscaled to grayscale images with a higher and constant resolution of 15 m, enhancing the flood predictions by considering small-scale topographic features, and then used as training data for the neural network. The many-to-one deep learning model predicts a single peak total water level in time at multiple locations in space using time series of the offshore astronomical tide and track parameters as inputs. We used the model to make probabilistic predictions of peak total water levels for observed and perturbed tracks of several historical storms that affected North Carolina.
We showed that the neural network performed well (with errors ranging from 8 to 43 cm) in predicting peak total water levels at nine locations in North Carolina. We applied the neural network to make probabilistic predictions of peak total water levels for observed and perturbed tracks of historical storms. For each storm, the neural network predicted at nine stations for 101 storm scenarios (the true/historical storm and 100 perturbations) in less than 10 seconds. The performance for the observed historical storms was similar to those obtained in process-based simulations, but with a significant gain in computational runtime.
Tomás Cuevas López defends MS Thesis
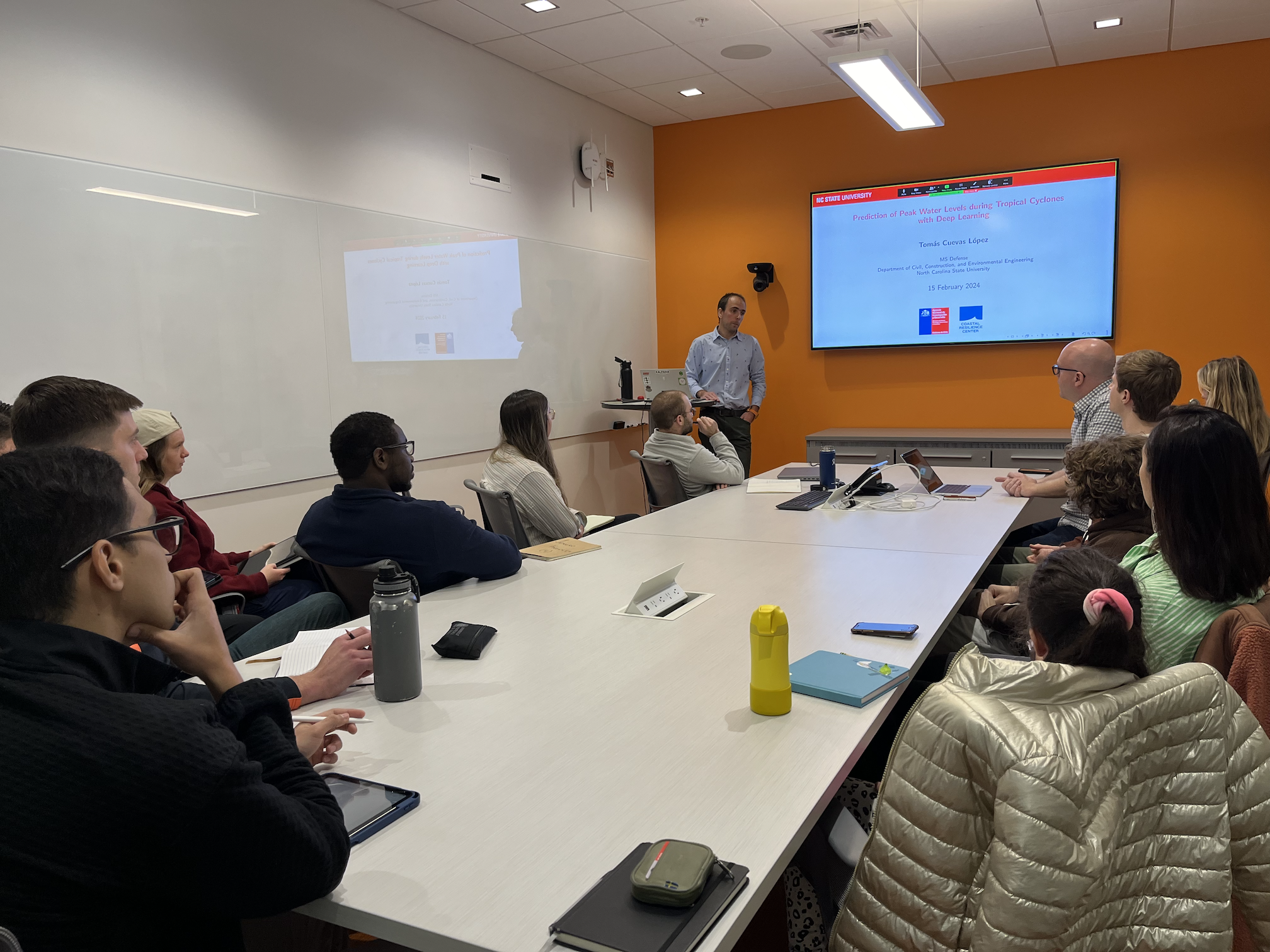
Tomás starts his thesis defense.
Thesis Defense: Tomás Cuevas López
North Carolina Center for Coastal Algae, People, and Environment
A Schnetzer, SM Belcher, BB Cutts, DR Obenour, T Ben-Horin, JC Dietrich, C Hoyo, NG Nelson, R Paerl. “North Carolina Center for Coastal Algae, People, and Environment (NC C-CAPE).” National Institutes of Health, National Institute of Environmental Health Sciences, Centers for Oceans and Human Health 4: Impacts of Climate Change on Oceans and Great Lakes, 2024/02/01 to 2029/01/31, $6,913,382 (Dietrich: $467,482).